Unleashing the Transformative Power of Spark Engineering in Modern Technology
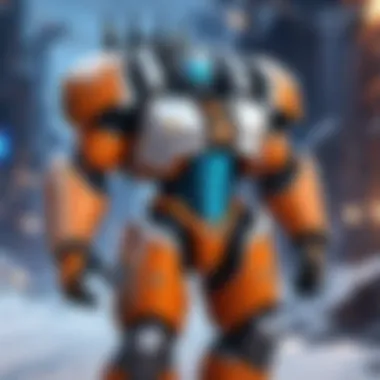
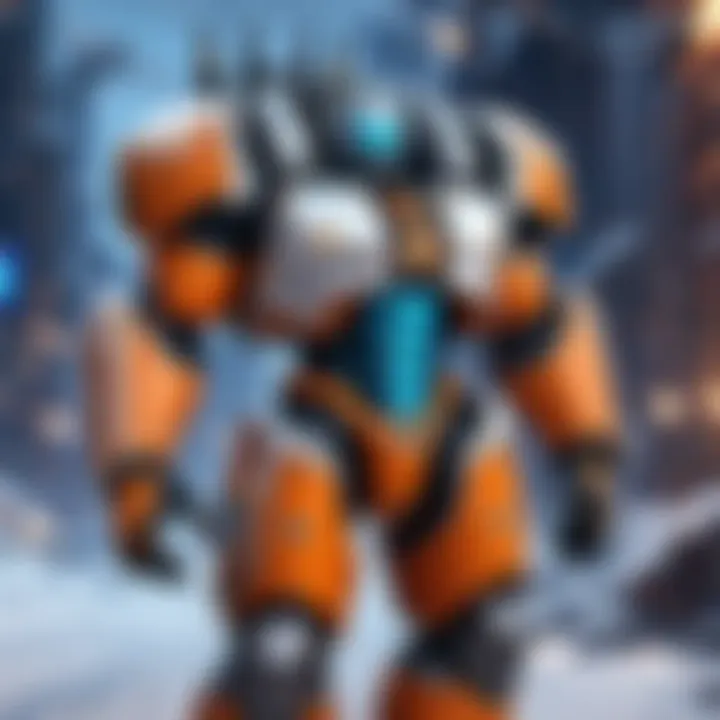
Game Updates and Patch Notes
- Overview of recent game updates by Blizzard
- Detailed breakdowon of patch notes and changes
- Analysis od impact on gameplay and community feeebask
This section critically explores the recent updates in the world of Blizzard games, providing a thorough evaluation of the changes introduced. From bug fixes to balance adjustments, Blizzard's continuous effort to enhance gameplay experience is analysed here in detail. Additionally, community reactions and feedback towards these updates are scrutinized to understand the impact on players and the overall gaming community.
Character Guides and Stratreges
- In-dpeth guides fo specific characters in Blizxard games
- Tips and tricks for mestering character abilities and playstyles
- Strategies for smechective gameplay and winningn strategdies
In this segment, the intricacies of character-specific guides and strategies within Blizzard games are meticulously examined. From understanding unique abilities to mastering complex playstyles, this section aims to equip players with in-depth knowledge and valuable insights on how to maximize their effectiveness in-game. Additionally, winning strategies and tips are provided to enhance overall gameplay and increase chances of success.
Comunnity News and Evenets
- Highlights of community events and touznaments related to Blizzard games
- Coverage of fan-made content and community creetions
- Updates on incominme Bliszard game releases antd special events
Delve into the vibrant world of community news and events surrounding Blizzard games in this section. From exciting tournaments to creative fan-made content, this segment highlights the diverse and engaging activities within the gaming community. Stay updated on upcoming Blizzard game releases and special events that cater to the enthusiastic player base, offering a comprehensive overview of the upcoming developments and happenings in the gaming realm.
E-sports and Comingpetitionle Scene
- Coveorage of e-sports tournments ad comprtitions for Blizzard games
- Playerl proceeds and intestiorhews with rbopdossimaln gamers
- Analpssis of compétie vet meeta and strategies usbwd in tournammentk
Immerse yourself in the thrilling world of e-sports tournaments and competitive matches within Blizzard games through this section. Comprehensive coverage of top-tier competitions, player profiles, and exclusive interviews with professional gamers offer a deep insight into the competitive gaming scene. Analysis of the competitive meta and strategic approaches employed during tournaments provide valuable information for both aspiring and seasoned competitors.
Fabn Twereies and Lori Discussions
- Interoretation of the storyline and lore in Blitzarrd games
- Tan theories andspeclauon onpqnc upcomigns game narratives
- Explronqtion ofshedib secerts and Eaasyer okld witl thin game modousing
Unravel the mysteries and depths of the intricate storyline and lore embedded within Blizzard games in this captivating section. From interpreting complex narratives to exploring fan theories and speculations on future game developments, this segment offers a journey into the rich storytelling elements of Blizzard games. Dive into the realms of hidden secrets, Easter eggs, and captivating lore discussions within the expansive game worlds, providing a holistic view of the immersive and captivating storytelling within Blizzard games.
Introduction to Spark Engineering
In the realm of modern technology, the advent of Spark Engineering has heralded a new era of innovation and efficiency. Spark Engineering serves as the bedrock upon which our digital advancements stand, propelling industries into uncharted territories of optimization and speed. Understanding the nuances of Spark Engineering is crucial for navigating the complexities of today's tech landscape. Its multifaceted applications span across varied sectors, offering solutions that redefine the concept of real-time data processing and computational prowess.
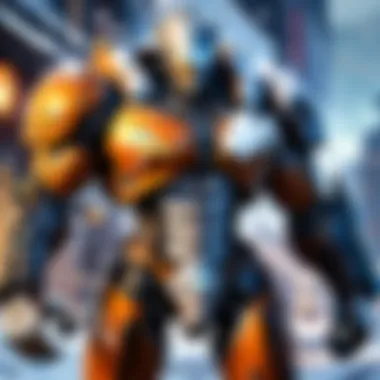
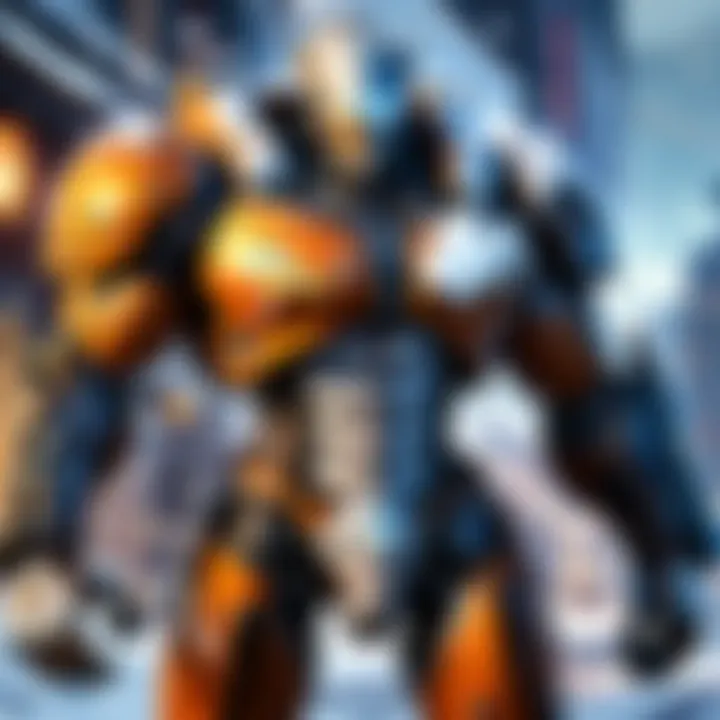
Understanding the Concept of Spark
Theoretical Framework of Spark
Delving into the Theoretical Framework of Spark unfurls a rich tapestry of computational intricacies. This framework forms the backbone of Spark's architecture, emphasizing parallel processing and distributed computing. One key characteristic of the Theoretical Framework of Spark lies in its ability to handle massive datasets with unparalleled speed and agility. Its unique feature lies in its fault-tolerant nature, ensuring reliable data processing even in the face of unexpected errors or failures. While incredibly potent, complexities may arise in optimizing resources efficiently within this framework.
Key Principles of Spark Technology
As we navigate the Key Principles of Spark Technology, we unearth fundamental pillars that support Spark's functionality. These principles outline Spark's approach to tackling data processing challenges, focusing on scalability and adaptability. A key characteristic of Spark's principles is its in-memory computing capability, enabling rapid data processing by storing intermediate results in memory. This makes Spark a popular choice for real-time analytics and iterative algorithms. However, while this feature enhances speed, it may also pose challenges in managing memory resources effectively.
Evolution of Spark Engineering
Pioneering Innovations in Spark Technology
Explore the Pioneering Innovations in Spark Technology that have shaped our current technological landscape. These innovations have propelled Spark into the forefront of data processing technologies, offering solutions that surpass traditional batch processing methods. One key characteristic of these innovations is their emphasis on streamlining complex algorithms and optimizing computational workflows. Their unique feature lies in their adaptability to diverse data types and sources, making Spark a versatile choice for various industries. However, integrating these innovations may require significant restructuring of existing systems and data pipelines.
Impact of Spark on Data Processing
The Impact of Spark on Data Processing cannot be overstated in the digital age. Spark's influence has revolutionized traditional data processing methodologies, ushering in an era of lightning-fast analytics and scalable computing. One key characteristic of Spark's impact lies in its ability to process data in near real-time, providing instant insights for informed decision-making. Its unique feature lies in its distributed computing model, empowering organizations to handle massive datasets with ease. Despite its advantages, incorporating Spark into data processing workflows may necessitate additional training and resource allocation.
Applications Across Industries
In the realm of modern technology, the significance of Spark Engineering transcends boundaries by playing a pivotal role in various industries. From data analytics to machine learning and cloud computing, Spark's applications across industries showcase its versatility and transformative capabilities. By harnessing Spark in data analytics, sectors can gain real-time data insights that redefine decision-making processes and drive business strategies towards optimal outcomes. The utilization of Spark in machine learning empowers organizations to optimize algorithm performance, leading to enhanced model accuracy and efficiency. Moreover, Spark's role in cloud computing offers scalability in cloud infrastructure, enabling businesses to dynamically adjust resources based on demand levels and ensuring efficient resource management. The seamless integration of Spark across industries underscores its profound impact on modern technological advancements.
Spark in Data Analytics
Real-Tmee Data Insights
Embarking on the journey of data analytics, companies harness Spark to delve deep into the realm of real-time data insights. By leveraging Spark's robust capabilities in processing vast amounts of data with unparalleled speed, organizations can extract actionable insights promptly. Referred to as 'Reeal-Tmee Data Insights' in this context (), this aspect entails the instantaneous analysis of data streams, allowing for agile decision-making and adaptive strategies. The key characteristic of 'Reeal-Tmee Data Insights' lies in its ability to deliver up-to-the-second information, facilitating real-time adjustments to operational processes. Despite its benefits of quick responsiveness and enhanced agility, challenges may arise in managing the influx of real-time data and ensuring data accuracy within accelerated timelines.
Enhanced Pexdictiv Analytics
Moving ahead in the data analytics journey, 'Enhanced Predicticve Analytics' () emerges as a cornerstone in leveraging predictive modeling for future insights. This facet of Spark's data analytics capabilities focuses on enhancing predictive accuracy and forecasting potential outcomes with precision. The unique feature of 'Enhanced Pexdictiv Analytics' revolves around its iterative model refinement process, allowing for continuous learning and adaptation in predictive algorithms. While this approach offers significant advantages in predictive accuracy and strategic planning, complexities may arise in handling vast datasets and maintaining model relevance over time.

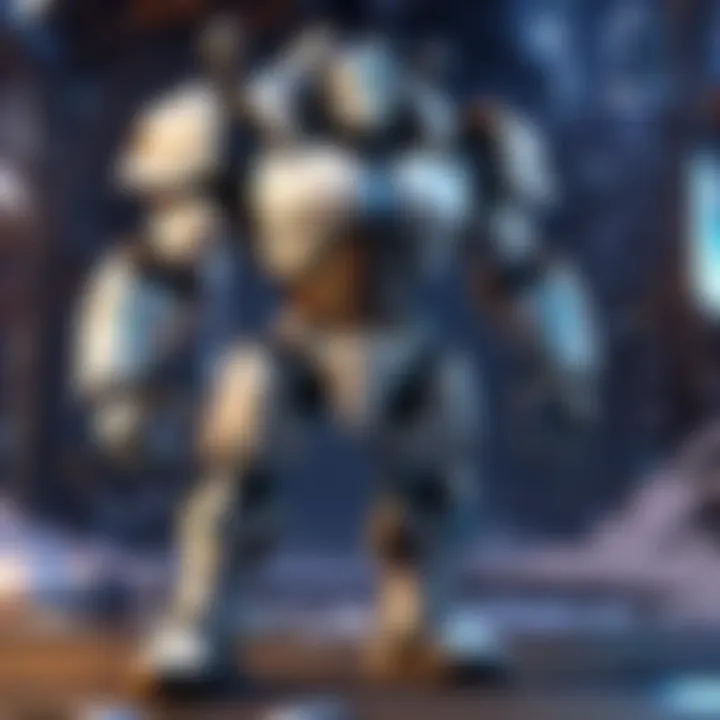
Utilizing Spark in Machine Learning
Optimiziing Algorithm Performance
Within the domain of machine learning, Spark facilitates the optimization of algorithm performance to elevate model efficiency () and accuracy. By streamlining complex algorithms through distributed processing, Spark empowers machine learning tasks to achieve higher computational speeds and enhanced predictive capabilities. The key characteristic of 'Optimiziing Algorithm Performance' lies in its ability to parallelize computations across clusters, expediting model training processes and supporting large-scale data analysis. Despite its advantages in accelerating model training and improving algorithm efficiency, challenges may arise in optimizing cluster configurations and addressing latency issues in distributed computing environments.
Facilitating Model Trianing
Continuing the journey through machine learning applications, 'Facilitating Model Trianing' () emerges as a critical aspect of utilizing Spark for model development. This component focuses on streamlining the model training process by optimizing resource allocation and enhancing training workflows. The unique feature of 'Facilitating Model Trianing' lies in its ability to automate repetitive tasks in model development, increasing operational efficiency and reducing manual intervention. While this approach offers advantages in minimizing training times and improving model scalability, complexities may arise in balancing computational resources and managing data dependencies effectively.
Spark's Roel in Cloud Computing
Scalaility in Cloud Infrastructure
Amidst the landscape of cloud computing, Spark contributes to enhancing scalability in cloud infrastructure by enabling dynamic resource allocation based on workload demands. The key characteristic of 'Scalaility in Cloud Infrastructure' () lies in its ability to scale processing capabilities horizontally, allowing for seamless expansion of computing resources as data volumes grow. This approach ensures optimal resource utilization and elasticity in cloud environments, catering to fluctuating computational needs. Despite the advantages of scalable infrastructure and on-demand resource provisioning, challenges may surface in optimizing resource utilization efficiency and cost management in dynamically scaling environments.
Effecided Resource Management
Furthermore, Spark plays a crucial role in fostering efficient resource management within cloud computing ecosystems, ensuring optimal resource allocation and utilization. 'Effecided Resource Management' () emphasizes the importance of strategic resource planning and allocation to minimize wastage and maximize performance efficiency. The unique feature of 'Effecided Resource Management' lies in its adaptive resource allocation algorithms, dynamically adjusting resource allocation based on application requirements. While this approach offers benefits in cost optimization and performance enhancement, complexities may arise in fine-tuning resource allocation strategies and balancing resource utilization across diverse workloads.
Impact on Technological Advancements
Enhancing Computational Speed
Innovations in Parallel Processing
When it comes to the realm of Innovations in Parallel Processing within the domain of Spark Engineering, we encounter a game-changing facet of technology. The ability to process multiple tasks concurrently is a hallmark of parallel processing, enabling substantial acceleration of computational speed. By tackling tasks simultaneously, Spark's innovations in this sphere alleviate bottlenecks and expedite data processing pipelines. The underlying advantage of parallel processing lies in its capacity to boost efficiency and scalability, making it a quintessential choice for handling vast datasets with precision and agility. One of the unique features of Innovations in Parallel Processing is its seamless integration with distributed computing frameworks, enhancing fault tolerance and reliability in complex data processing scenarios.
Performance Optimization Techniques
Within the realm of Performance Optimization Techniques lies a treasure trove of strategies aimed at fine-tuning Spark's computational capabilities. By leveraging these techniques, Spark Engineering achieves peak performance levels, optimizing resource utilization and minimizing processing delays. The key characteristic of Performance Optimization Techniques is their versatility in enhancing algorithm efficiency and data throughput. This facet of Spark technology stands out as a popular choice for organizations seeking to maximize computational resources effectively. However, it is essential to note that while Performance Optimization Techniques offer substantial performance gains, they may require meticulous implementation and fine-tuning to align seamlessly with specific use cases.
Revolutionizing Big Data Management
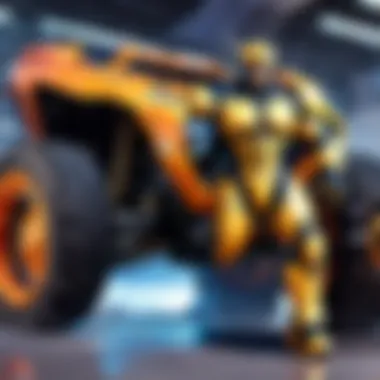
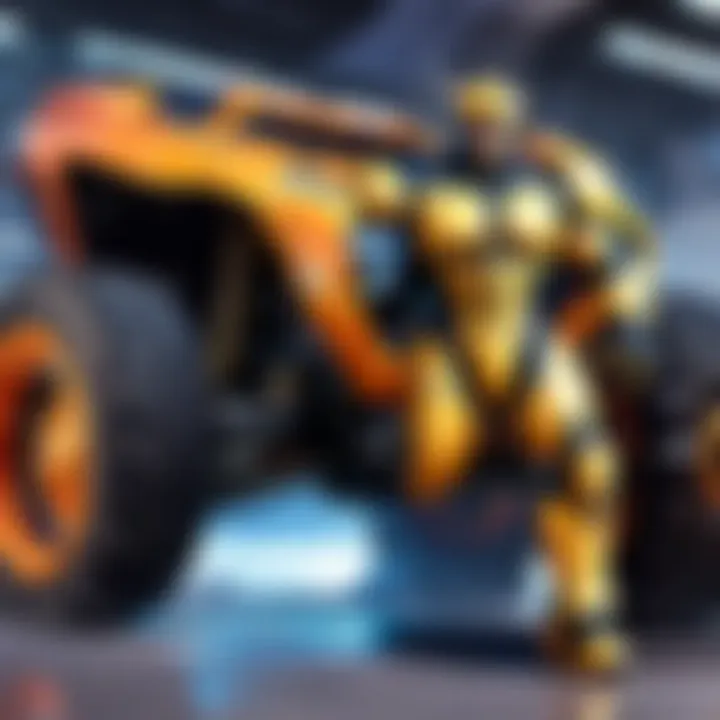
Scalable Data Processing Solutions
When delving into the realm of Scalable Data Processing Solutions within the context of Spark Engineering, we encounter a transformative approach to managing vast amounts of data effectively. The key characteristic of these solutions lies in their ability to scale seamlessly with growing data volumes, ensuring smooth and efficient processing. In our article exploring Spark Engineering, these solutions emerge as a beneficial choice due to their robust architecture and adaptability to dynamic data demands. One of the unique features of Scalable Data Processing Solutions is their capacity to operate across distributed computing environments, enabling enterprises to harness the power of big data with ease and efficiency.
Data Warehousing Strategies
Within the sphere of Data Warehousing Strategies, we uncover a strategic approach to data management that complements the overarching goal of Spark Engineering in modern technology. The key characteristic of these strategies is their focus on storing and retrieving data in a structured and efficient manner, facilitating swift access to critical information. In the context of this article, Data Warehousing Strategies stand out as a popular choice for organizations seeking to centralize their data assets and streamline analytical processes. One of the unique features of Data Warehousing Strategies is their integration with business intelligence tools, empowering decision-makers with valuable insights to drive strategic initiatives effectively.
Fostering IoT Development
Integration with Internet of Things
In exploring the facet of Integration with Internet of Things within Spark Engineering, we uncover a symbiotic relationship that propels IoT development to new heights. The key characteristic of this integration is its ability to seamlessly connect IoT devices to Spark clusters, enabling real-time data processing and analysis. In the narrative of our article, Integration with Internet of Things emerges as a beneficial choice for organizations looking to harness the potential of IoT in conjunction with Spark's robust infrastructure. A unique feature of this integration is its capacity to ingest and process sensor data in real-time, paving the way for innovative IoT applications across diverse industries.
Real-World Applications of IoT
As we navigate the realm of Real-World Applications of IoT within the context of Spark Engineering, we uncover practical use cases that exemplify the impact of IoT integration with Spark technology. The key characteristic of these applications is their ability to transform raw sensor data into actionable insights, driving informed decision-making processes. In our detailed exploration, Real-World Applications of IoT emerge as a popular choice due to their tangible impact on enhancing operational efficiency and unlocking new revenue streams for businesses. A unique feature of these applications is their versatility in supporting various IoT use cases, ranging from smart cities to industrial automation, underscoring the transformative potential of IoT technology when combined with the computational power of Spark Engineering.
Challenges and Future Prospects
Addressing Complexity in Spark Implementation
Optimizing Resource Allocation
Addressing the intricacies of resource allocation within the realm of Spark implementation is paramount for maximizing efficiency and performance. Optimizing how resources are assigned and utilized can significantly impact the success of Spark projects. By streamlining resource allocation processes, organizations can ensure that computational resources are allocated optimally to tasks, thereby enhancing overall system performance. The key characteristic of optimizing resource allocation lies in its ability to match computing resources with specific task requirements in a dynamic and scalable manner. This approach ensures that each task receives the necessary resources to execute effectively, leading to improved throughput and reduced latency. Despite its benefits, challenges may arise in balancing resource allocation, requiring careful monitoring and adjustment to maintain optimal system performance.
Enhancing Fault Tolerance Mechanisms
Enhancing fault tolerance mechanisms is a critical aspect of ensuring the reliability and robustness of Spark implementations. By fortifying systems against failures and errors, organizations can mitigate risks and prevent catastrophic data loss. The key characteristic of fault tolerance mechanisms in Spark lies in their ability to detect and handle faults seamlessly, ensuring uninterrupted operation even in the face of unexpected events. This proactive approach enhances the stability and resilience of Spark applications, safeguarding against potential disruptions and ensuring continuous data processing. While the integration of fault tolerance mechanisms offers significant advantages in maintaining system integrity, it also introduces complexities that require careful consideration. Balancing the performance impact of fault tolerance measures with their benefits is essential to achieve an optimal level of system reliability.
Exploring Innovations in Spark Technology
Advancements in Spark Ecosystem
Exploring advancements in the Spark ecosystem unveils a wide range of improvements that elevate the capabilities of Spark technology. These advancements contribute to enhancing the scalability, performance, and reliability of Spark-based solutions, positioning them as leading choices in the digital landscape. The key characteristic of advancements in the Spark ecosystem is their focus on refining core functionalities, expanding compatibility, and integrating cutting-edge features to meet evolving industry demands. By embracing these advancements, organizations can leverage the latest innovations in Spark technology to drive efficiency and competitiveness. However, while the advancements bring immense benefits, considerations must be made regarding compatibility, training, and integration efforts to fully harness their potential without disrupting existing systems.
Integration with Emerging Technologies
The integration of Spark technology with emerging technologies presents exciting possibilities for driving synergies and unlocking new capabilities. By aligning Spark with advancements in fields such as artificial intelligence, blockchain, and Internet of Things, organizations can create powerful solutions that merge data processing capabilities with innovative functionalities. The key characteristic of integrating Spark with emerging technologies is the fusion of diverse technical domains to create holistic systems that deliver enhanced performance and insights. This strategic integration opens doors to novel use cases and applications that transcend traditional boundaries, paving the way for groundbreaking technological solutions. While the advantages of integration with emerging technologies are clear, the challenges lie in managing complex ecosystems, ensuring interoperability, and navigating regulatory frameworks to maximize the value derived from these innovative partnerships.
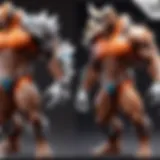
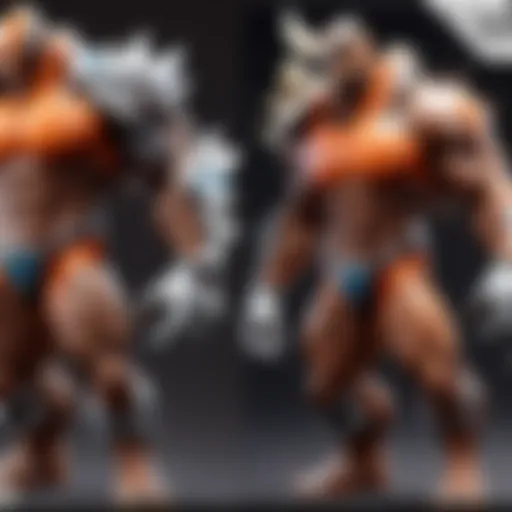